AI, ML are Pushing Media QC and Monitoring to the Next Level
Ensuring a high-quality video experience on every screen is essential if broadcasters want to keep viewers satisfied
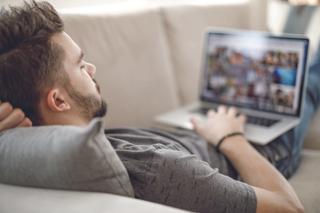
Over the years, the complexity of video preparation and delivery has increased dramatically. First, the industry witnessed the move from tape to file-based workflows, followed by the transition from analog to digital. New formats and standards have also emerged, adding to the complexity of video delivery.
Aside from these technology transformations, consumer viewing habits are shifting. Today’s viewers prefer OTT media services, with 76% of U.S. households subscribing to OTT services compared with 62% for traditional pay-TV, according to the latest research from Parks Associates. As broadcasters deliver a higher volume of content to a wider range of screens and global audiences, additional errors are being introduced into the workflow, potentially affecting video and audio quality.
Recent advancements in automated media quality control and monitoring systems are helping broadcasters deliver error-free video and audio on every screen. In particular, innovations in machine learning and artificial intelligence are pushing media QC and monitoring to the next level, increasing the accuracy and consistency of certain media tasks, including content classification, content categorization, lip sync checks and more.
MEDIA QC AND MONITORING IS EVOLVING
In the early stages of media QC and monitoring, automated systems were limited to simple tasks, such as checking the correctness of audio/video technical parameters, including resolution, frame rate, bitrate, content structure and container parameters.
Since then, media QC and monitoring has evolved. Today, broadcasters can check for perceptual errors using computer vision and standard audio processing techniques. These checks include interlace artifacts, defective pixels, dropouts, visual text recognition, compression and ghosting artifacts, loudness and language detection.
With the rise of ML and its success in completing tasks such as content classification and object detection, the scope of media QC and monitoring has expanded. Now broadcasters are using advanced ML techniques capable of semantically understanding content for the purpose of content moderation, content classification, indexing and description generation. Let’s look at a few of the specific media applications that can be optimized with ML and AI technologies.
SPEEDING UP CONTENT COMPLIANCE WITH ML
Monitoring and altering content in order to conform to different rules and regulations is one application that can greatly benefit from ML. Broadcasters must comply with a wide range of rules and regulations, which can vary from one region to another.
Traditionally, broadcasters have maintained a pool of human moderators to manually filter content for regulatory compliance. Under a typical manual workflow, content is passed through multiple stages of review. If a review fails at any stage, the content goes back for editing. Manual content QC and monitoring is expensive, time-consuming and inaccurate. With so many global and regional aspects of content moderation, it is almost impossible for humans to carry out the job with 100% accuracy.
By automating this process, broadcasters can eliminate the limitations of manual content moderation, including the inability for people to memorize a significant number of visual symbols and the possibility for human error. With an automated QC and monitoring workflow, broadcasters can more rapidly and accurately check content for the presence of brand names, hate symbols, alcohol, violence, celebrity faces, vulgar speech captions and religious symbols.
When using an automated system powered by ML, computer vision techniques and computer algorithms, the benefits are even greater. ML-based systems can handle huge and multiple content classification check lists without any major performance limitations, driving broadcast workflow efficiencies.
However, it’s important to note that while current ML solutions are sophisticated and may be combined to create broader applications, they lack the real-world knowledge and human experience needed to create valid and acceptable outcomes on their own. Human input is still required to confirm the validity of patterns and help machines refine the result. Such human interactions are likely to define ML uses in the media industry for the foreseeable future.
ENSURING SUPERIOR QUALITY CAPTIONS WITH ML
Checking for the presence and accuracy of captions is another application area where ML has proven to be very effective. ML can be used to automatically generate captions where they are not present in the content, check the alignment between captions and audio, and check the correctness of the captions against the spoken audio. In addition, ML simplifies the identification of speakers in audio, ensuring that the correct punctuations are placed in captions.
Ultimately, with ML, broadcasters can expedite the caption creation and verification processes for both live and VOD content, while ensuring that when content is delivered in multiple video quality levels within OTT video streams, the captions maintain a high quality.
Over the last decade, automatic speech recognition engines have achieved extremely high accuracy, up to 85%, via ML. Still, automatic speech engines face several challenges, such as robustness issues in noisy environments, the ability to handle variable accents, problems when multiple speakers are talking at the same time, and difficulty with kids’ voices (due to a lack of data to train ML models).
Keeping humans in the loop is imperative to resolve these challenges. By combining cutting-edge ML and automatic speech recognition technology with a manual review process, broadcasters can bring increased simplicity and cost savings to the creation, management and delivery of captions for traditional TV and video streaming.
ELIMINATING AV LIP SYNC ISSUES WITH ML
Synchronization between audio and video is a common issue today. Leveraging image processing and ML technology and deep neural networks, broadcasters can automatically detect audio and video sync errors. ML offers a faster and more precise approach to detecting audio lead and lag issues in media content, compared with the traditional approach of manually checking for lip sync errors. This allows broadcasters to provide a high quality of experience to viewers (QoE).
Through the power of ML, broadcasters can perform facial detection, facial tracking, lip detection, lip activity detection and speech identification. With an ML-based lip sync solution, typically one module uses video to extract faces and track lip movement. A second module uses audio to extract audio features and a third ML module matches the movements with the audio features. Using this technique, it is possible to detect even one frame of synchronization issues.
CONCLUSION
The amount of content that broadcasters are delivering across the globe is massive. Ensuring a high-quality video experience on every screen is essential if broadcasters want to keep viewers satisfied. With automated QC and monitoring solutions featuring ML and AI technology, broadcasters are better placed to quickly and more accurately comply with industry and government regulations, deliver high-quality captions, classify and categorize content and eliminate lip sync issues.
Anupama Anantharaman is vice president, Product Management, at Interra Systems.
Get the TV Tech Newsletter
The professional video industry's #1 source for news, trends and product and tech information. Sign up below.
Anupama Anantharaman, Vice President, Product Management at Interra Systems, is a seasoned professional in the digital TV industry. Based in Silicon Valley, California, Anupama has more than two decades of experience in video compression, transmission, and OTT streaming. Her journey began as a software engineer at Compression Labs – a trailblazer in MPEG-based digital video – where she contributed significantly to the development of videoconferencing systems. Over the years, Anupama has transitioned into roles encompassing product management and business development, primarily concentrating on internet-based video communications. At Interra Systems, she spearheads the product marketing team, overseeing activities ranging from product definitions and launches to strategic selling and fostering technology alliances. Her focus lies in enhancing video quality control, monitoring, and analyzer products to meet the evolving demands of the media and entertainment industry.