AI-Generated Audio: The Government, Media and Doomsayers
While AI/ML is not new in media production, new developments bring it to the forefront
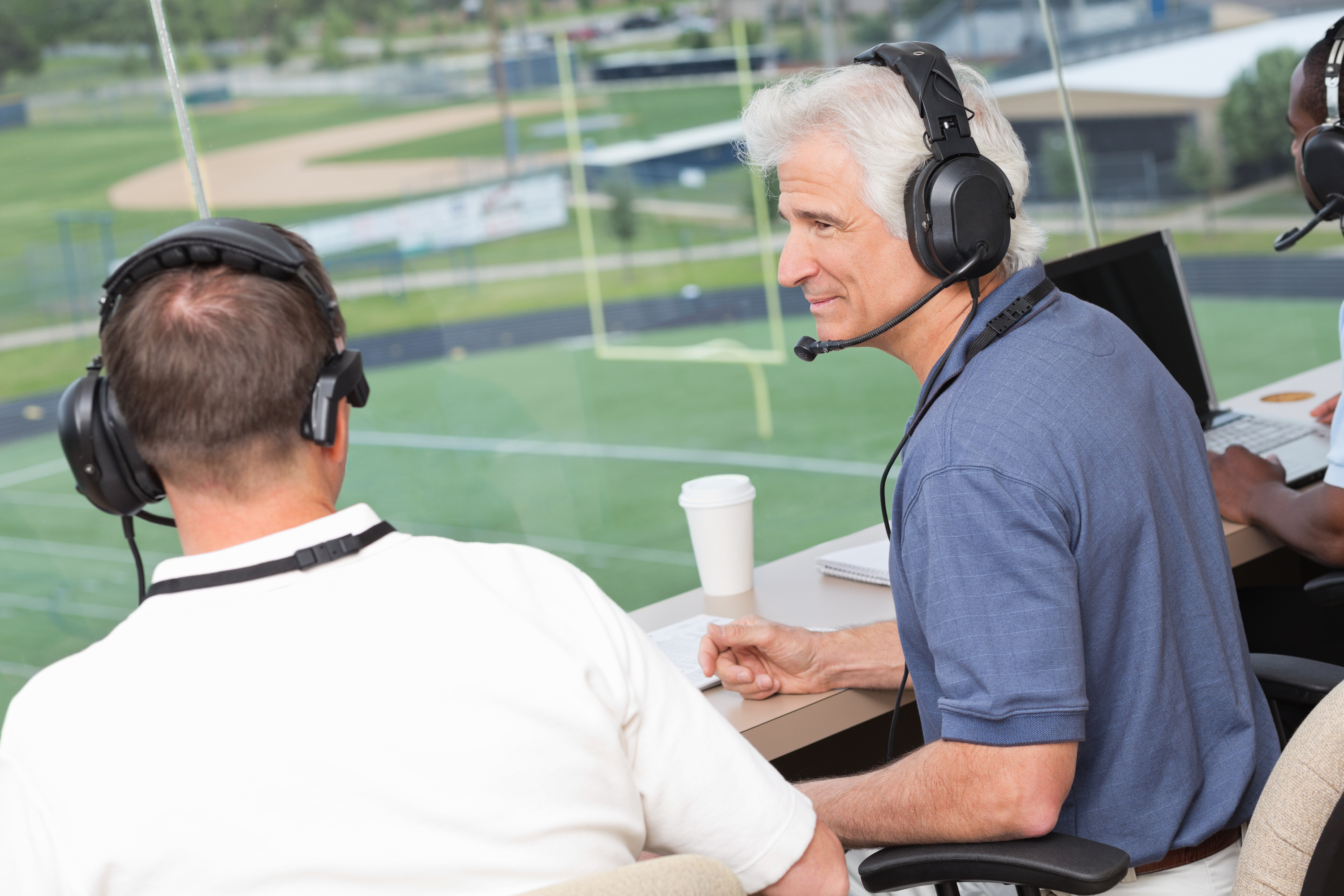
This year we have heard the terms "artificial intelligence," "machine learning" and "deep fakes" tossed around frequently, particularly since Wall Street forecasters have discovered AI as a financial evaluation metric for a tech company’s future performance. Companies that are known to support broadcasting and media applications such as Microsoft (ChatGPT), Adobe, IBM (Watson), NVIDIA and Soundhound AI, all promote possibilities with the continued development of this technology.
After some research, I am still trying to understand what seems to be an evolving definition of AI, but I have no doubt that smart/fast computers will significantly impact broadcasters and media companies.
AI seems to encompass a bundle of operations like machine learning and computer-created deep fakes, dependent upon analyzing vast amounts of data to predict, create and deliver a desirable outcome. Content companies such as Netflix have benefitted from AI with computer-generated programing recommendations based on accumulated information of a person’s search and viewing habits.
Music, Hollywood Scripts
Computer jocks have used AI to develop complex algorithms to create art, music, even dialog for movie and TV scripts, (music, for instance, is pretty repetitive—you can only imagine how many hit songs are based on three chords/notes and a rhyming dictionary). I also think that there is no reason not to believe that ChatGPT could write a Hollywood script. Remember, the last Hollywood writers strike gave us “unscripted” reality TV—often a waste of electronic transmission time and electricity.
Since machine learning is data-driven, it is dependent on the accumulation of more and more data samples to improve the results. Constant sampling only improves the outcome and has been particularly effective with the AI sub-field of “deep fakes.” We think of deep fakes as replicating a person or object’s face or voice onto another, but deep fakes are as old as TV itself—background sound effects such as laugh and clap tracks, even coconuts used for horse hooves were intended to imitate reality and fool the gullible listener with the magic of radio and television.
Clearly machine learning has applications in broadcasting.
There is no question that HBS—Host Broadcast Services, the production company for World Cup Football—used fast computers to make possible accurate microphone selection and mixing possible. Lawo worked with HBS to develop a mixing system that takes data of the ball position and translates that into an algorithm that captures the best possible sound from the best microphone or combination of microphones, plus determines the level to mix and blend the microphones together. Tracking the ball is done optically and in a sport like football, the focus of the game is the ball; basically, you tell the computer to follow the ball.
Is this artificial intelligence? I would say more “deep learning.” Another sophisticated automated mixing algorithm, “Spatial Automated Live Sports Audio” or SALSA, was developed by my friends Rob Oldfield and Ben Shirley.
“In our case we are primarily using deep learning to automatically recognize sound events in broadcast microphones so we can automate and enhance the on-field mixing,” Oldfield said. “We are increasing what we are doing with AI beyond this though, and are now looking at crowd and commentary sentiment analysis so we can generate metadata for other parts of the broadcast chain, for example, automatic highlight generation.
“It occurs to me that there is a lot of data from the microphones at an event (not just sound capture),” he added. “I think this has been overlooked in the past, but with the increased power of deep learning and AI we are in a better place to fully use the microphones as ‘data gatherers’ and audio can add value to all parts of the broadcast and fan experience.”
AI-Assisted Audio
Now let’s follow the flow for typical sport coverage and look at the advanced possibilities of AI—machine learning for sports coverage. Camera robotics has been around for a while and there is no reason the cameras and audio cannot follow the electronic commands of a computer that is following play action.
"With the increased power of deep learning and AI we are in a better place to fully use the microphones as ‘data gatherers’ and audio can add value to all parts of the broadcast and fan experience.”
Rob Oldfield
AI comes into play when a computer analyzes the switching patterns and compares the director’s commands to the position of the ball within the field of view of the broadcast cameras. The computer archives the director’s selection and patterns for future progressive learning and within a short period of time, repetitions will be detected, examined and programmed into event cycles to take over the direction of the cameras and audio.
AI-assisted audio coverage/production could include speech interpretation and synthesis such as AI-driven subtitles, but could AI mimic a play-by-play or color commentator?
Consider this: The computer can learn styles from “real life” commentators and further learn how to filter information from the cameras to match the visual action and build a reference library of players or actors. The “speech” computer can ingest all the data and artificially create the commentary track and even mimic certain styles and accents.
Speech synthesis has been around awhile and with the addition of faster computers and machine learning it becomes conceivable that you can create droid commentators that interpret/present the play-by-play action and side stories to complete the entire experience.
Sound analysis has been common practice, however artificial intelligence would be good at evaluating patterns and picking the best choice(s) for a replay from a set of indicators. For example, a very loud, sudden burst of crowd (sharp attack) with a long sustain is probably a good indication of a goal.
The vocal inflections of the crowd—sustained screaming as opposed to a sigh of dismay that dies out quickly is another valuable and identifiable metric. From these simple learning indicators, the computer within a dozen repetitions will be able to accurately predict a good highlight moment.
Clearly media production will benefit from computer augmentation and machine learning simply because of the amount of content that needs to be generated. Media entertainment cuts across a variety of viewing and listening options including small or large screens, even goggles with anything from immersive sound to earbuds and an adaptive learning algorithm such as AI can only contribute to the elevated experience.
It all impacts TV technology, particularly since we work in a deeply computerized world. Where do you fit in this brave new world.
Get the TV Tech Newsletter
The professional video industry's #1 source for news, trends and product and tech information. Sign up below.
Dennis Baxter has spent over 35 years in live broadcasting contributing to hundreds of live events including sound design for nine Olympic Games. He has earned multiple Emmy Awards and is the author of “A Practical Guide to Television Sound Engineering,” published in both English and Chinese. His current book about immersive sound practices and production will be available in 2022. He can be reached at dbaxter@dennisbaxtersound.com or at www.dennisbaxtersound.com.